Recently, a research paper of Associate Professor Wang Jian (College of Science, China University of Petroleum, East China)’s team has been published by <<IEEE Transactions on Cybernetics>>, which is a top journal in the field of cybernetics and artificial intelligence. This paper, titled by “Weight Noise Injection-Based MLPs With Group Lasso Penalty: Asymptotic Convergence and Application to Node Pruning”, has obtained new research results in neural network fault-tolerant learning theory and structural optimization research. It is resulting from Jian’s collaboration with Professor Nikhil R. Pal (Indian Statistical Institute, Member of the Indian National Science Academy, IEEE Fellow). Associate Professor Wang Jian is the first author of the paper, and China University of Petroleum (East China) is the first signed unit.
The research was supported in part by the National Natural Science Foundation of China under Grant 6130507, in part by the Natural Science Foundation of Shandong Province under Grant ZR2015AL014 and Grant ZR201709220208, and in part by the Fundamental Research Funds for the Central Universities under Grant 15CX08011A and Grant 18CX02036A.

Neural networks are simple simulations of the human brain's nervous system and are widely studied for their ability to handle nonlinear problems. The use of neural networks for fault-tolerant control of dynamic systems is an important part of modern fault-tolerant control technology. These methods have been successfully applied in many engineering fields. The existing researches mainly consider adding L2 regularization to improve the fault-tolerant learning algorithm, design the objective function and analyze the asymptotic convergence. However, because the L2 regularization usually does notproduce sparse solutions, it cannot get good results when dealing with variable selection, feature extraction and structural optimization, which limits its application in neural networks.
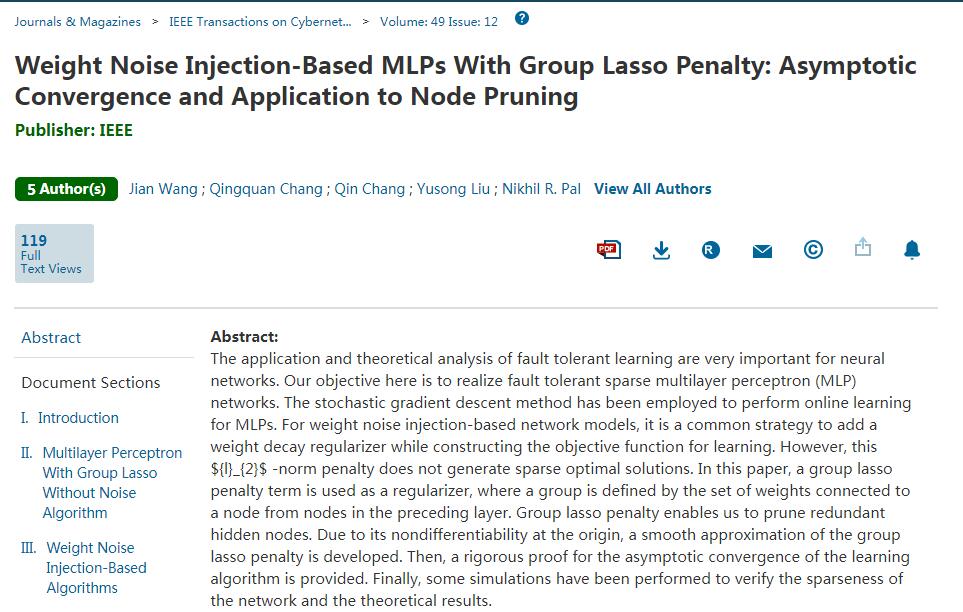
In response to the above problems and from the perspective of network model design, Jian's team added the Group Lasso regularizer while considering additive noise and multiplicative noise during the training process. Experimental results show that the designed fault-tolerant learning algorithm is robust to both the above typical noises. Compared to the L2 regularization, the Group Lasso regularization is more likely to produce sparse solutions. However, its non-convex and non-smooth characteristics tend to cause oscillations during the training process. The team effectively avoids the singularity problem by means of smooth approximation and rigorously proves the asymptotic convergence of the designed learning model. This approach provides technical support and theoretical guidance for the design of the optimization model for fault-tolerant learning structure.
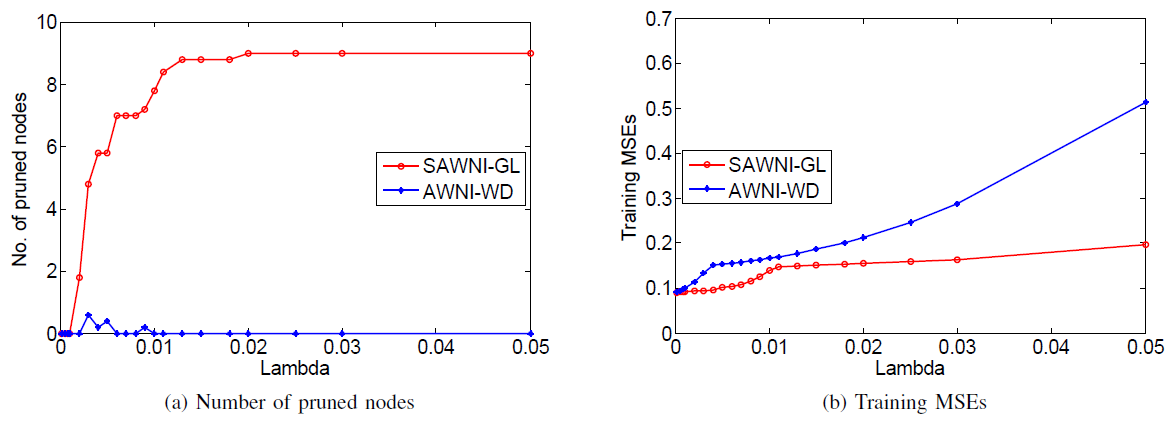
The reviewers believe that the research results have a good application and promotion value in the design of fault-tolerant learning models, especially in deep learning to construct the simplest network structure.
<<IEEE Transactions on Cybernetics>> is founded in 1960 and has a high influence in the fields of automation and control systems, artificial intelligence and cybernetics. It has very strict requirements for the innovation of the research content. The latest impact factor of the journal is 10.387, which makes it belong to the SCI TOP Journals.
Full text link: https://ieeexplore.ieee.org/document/8561200?denied=
(Editor: Xie Xuetao, Chang Qin)